- Diseases
- Acoustic Neuroma (14)
- Adrenal Gland Tumor (24)
- Anal Cancer (66)
- Anemia (2)
- Appendix Cancer (16)
- Bile Duct Cancer (28)
- Bladder Cancer (68)
- Brain Metastases (28)
- Brain Tumor (230)
- Breast Cancer (716)
- Breast Implant-Associated Anaplastic Large Cell Lymphoma (2)
- Cancer of Unknown Primary (4)
- Carcinoid Tumor (8)
- Cervical Cancer (154)
- Colon Cancer (164)
- Colorectal Cancer (110)
- Endocrine Tumor (4)
- Esophageal Cancer (42)
- Eye Cancer (36)
- Fallopian Tube Cancer (6)
- Germ Cell Tumor (4)
- Gestational Trophoblastic Disease (2)
- Head and Neck Cancer (6)
- Kidney Cancer (124)
- Leukemia (344)
- Liver Cancer (50)
- Lung Cancer (288)
- Lymphoma (284)
- Mesothelioma (14)
- Metastasis (30)
- Multiple Myeloma (98)
- Myelodysplastic Syndrome (60)
- Myeloproliferative Neoplasm (4)
- Neuroendocrine Tumors (16)
- Oral Cancer (100)
- Ovarian Cancer (170)
- Pancreatic Cancer (166)
- Parathyroid Disease (2)
- Penile Cancer (14)
- Pituitary Tumor (6)
- Prostate Cancer (144)
- Rectal Cancer (58)
- Renal Medullary Carcinoma (6)
- Salivary Gland Cancer (14)
- Sarcoma (236)
- Skin Cancer (294)
- Skull Base Tumors (56)
- Spinal Tumor (12)
- Stomach Cancer (60)
- Testicular Cancer (28)
- Throat Cancer (90)
- Thymoma (6)
- Thyroid Cancer (98)
- Tonsil Cancer (30)
- Uterine Cancer (78)
- Vaginal Cancer (14)
- Vulvar Cancer (18)
- Cancer Topic
- Adolescent and Young Adult Cancer Issues (20)
- Advance Care Planning (10)
- Biostatistics (2)
- Blood Donation (18)
- Bone Health (8)
- COVID-19 (362)
- Cancer Recurrence (120)
- Childhood Cancer Issues (120)
- Clinical Trials (624)
- Complementary Integrative Medicine (24)
- Cytogenetics (2)
- DNA Methylation (4)
- Diagnosis (230)
- Epigenetics (6)
- Fertility (62)
- Follow-up Guidelines (2)
- Health Disparities (14)
- Hereditary Cancer Syndromes (122)
- Immunology (18)
- Li-Fraumeni Syndrome (8)
- Mental Health (118)
- Molecular Diagnostics (8)
- Pain Management (62)
- Palliative Care (8)
- Pathology (10)
- Physical Therapy (18)
- Pregnancy (18)
- Prevention (892)
- Research (390)
- Second Opinion (74)
- Sexuality (16)
- Side Effects (604)
- Sleep Disorders (10)
- Stem Cell Transplantation Cellular Therapy (216)
- Support (404)
- Survivorship (322)
- Symptoms (184)
- Treatment (1772)
4 questions for mathematical oncologist Heiko Enderling, Ph.D.
3 minute read | Published January 28, 2025
Medically Reviewed | Last reviewed by Heiko Enderling, Ph.D., on January 28, 2025
For Heiko Enderling, Ph.D., the start of a question to solve a problem often begins with a pen on a paper napkin or chalk on a chalkboard. As a mathematical oncologist, he serves as the bridge between computational tools and data to clinical providers wanting to provide more data-driven personalized therapy recommendations for patients. The mathematical or computational models he creates can be applied to real cancer treatment scenarios to predict response to therapy for clinicians and patients to use in clinical decision-making.
How did you get your start as a cancer researcher?
While studying computer science as an undergrad, I interned as a programmer at a university math department, which inspired me to get my Ph.D. in mathematical oncology. I focused my fellowship on radiation modeling because my mother had had terrible radiation therapy side effects. I wanted to explore using data science to make patient-specific predictions to help improve treatment and our understanding of cancer.
What is unique about doing research here at MD Anderson?
I’m a data scientist working in a clinical department. I’m ‘in the room’ with clinical faculty as they discuss challenges. This means I hear directly from radiation oncologists the questions that need to be answered to help them in their clinical decision-making.
How would you describe your research with digital twins?
A digital twin is a virtual representation of a patient comprised of integrated models that show the patient's and tumor's physical characteristics in real-time. The computer models are updated using data from the real-world patient, and the patient’s treatment is updated with the predictions from the computer model.
It’s like hurricane forecast models. Instead of reviewing satellite images and measuring air speed and water conditions, we review radiology scans and other clinical variables to be used as data in a computer model. Instead of predicting a storm’s trajectory, we predict how a patient will respond to treatment – and, if needed, we can change treatment to change the trajectory for an individual patient. Through the data, we learn from what went well and didn’t go well for past patients to improve the treatment for the next patient.
With radiation used to treat about 60% of patients, we have an incredible opportunity to make a significant impact on our patients. To do this we need more resources, clinical trials and data so we can translate these predictive tools to the clinic and scale rapidly. We have the vision, leadership, proof of principles and the start of a team to make it happen.
What about coming to MD Anderson excites you the most?
The people at MD Anderson, and the idea of bringing data science to every decision through MD Anderson’s Institute for Data Science in Oncology. We’re building the infrastructure, environment and clinical workflows to make digital twins and personalized oncology a reality. Also, everybody is incredibly collaborative and laser-focused on one mission — to end cancer. Many of the research projects in my lab started with ideas and equations on a napkin with colleagues at restaurants and coffee shops. Here at MD Anderson, we are uniquely positioned to translate these ideas through the research pipeline into clinical impact.
Learn about MD Anderson’s Institute for Data Science in Oncology.
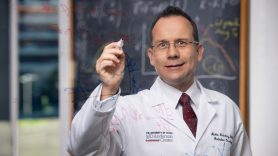
I wanted to explore data science to improve treatment and our understanding of cancer.
Heiko Enderling, Ph.D.
Researcher